© 2025 ALLCITY Network Inc.
All rights reserved.
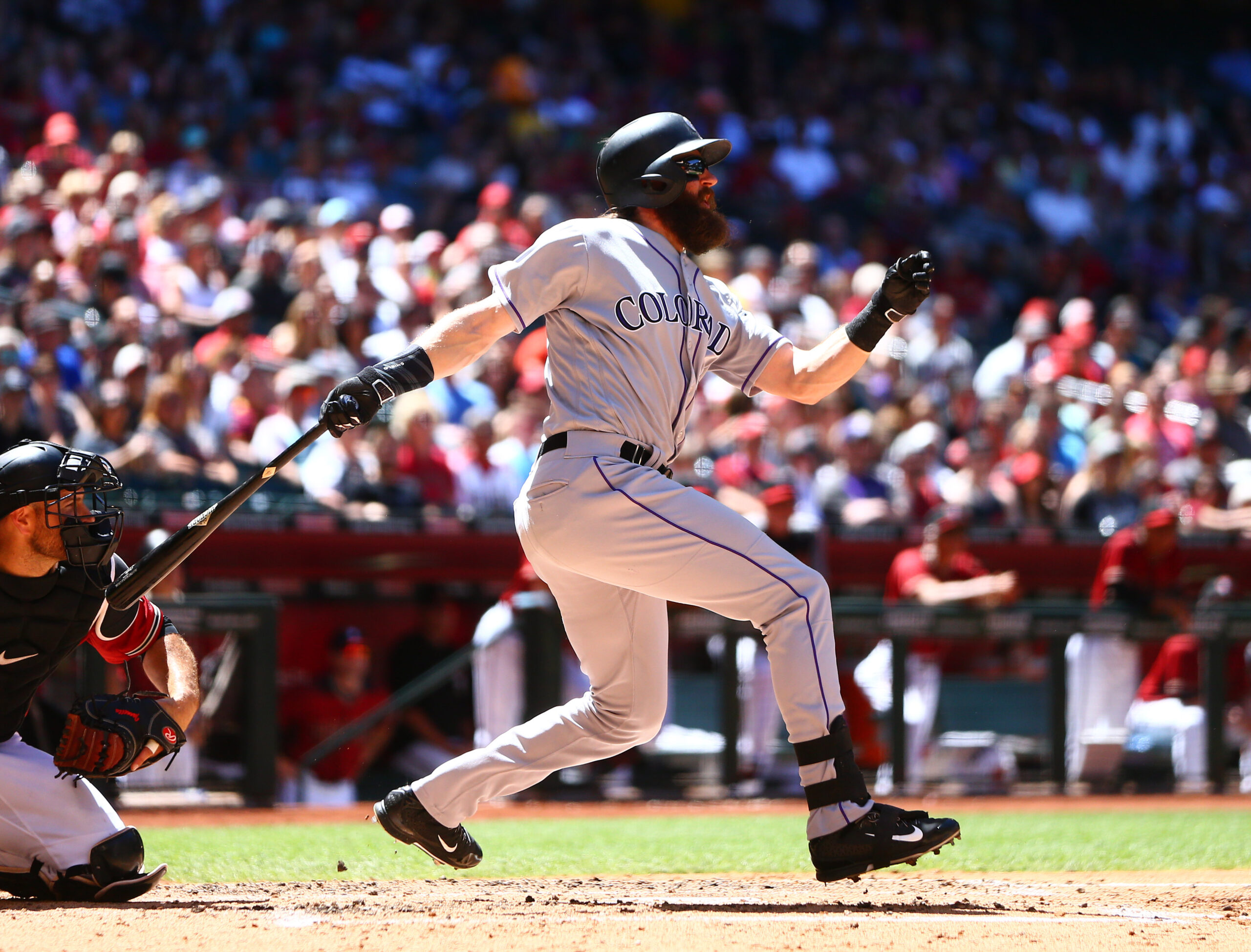
Wow. Just…. wow. I mean, I know the Rockies have historically been successful in April, but leading the NL at month’s end is something else. We are still only a month into the baseball season, however. Besides some trivial factoids to describe what has occurred thus far, what can we really conclude about this team’s performance thus far?
In answering such a question, we must be mindful of one very important aspect—sample sizes. We must first establish a threshold for sample size of each metric. Any samples greater than that of the established threshold can be used for meaningful analysis.
I’m not going to try and run the numbers on my own for this. There is plenty of precedent on the subject, beginning with Russell Carleton’s work all the way back in 2007 (Carleton used the alias ‘Pizza Cutter’ online prior to joining Baseball Prospectus).
Carleton established a simple method for establishing the necessary threshold for each individual metric, placing it at the point where the r value of the sample and it’s set of expected values equals 0.7. This means that Carleton believes a metric is reliable to use once the actual data and predicted data are at least 70% correlated. Carleton’s explains:
“In social science, we look for a magic number…and usually, the gold standard for reliability is .70…. Why am I obsessed with .70? Because a correlation of .70 means an R-squared of 49%. Anything north of .70 means that a majority of the variance (> 50%) is stable.”
In layman’s terms, a correlational value greater than 0.7 suggests at least half the variation in the data can explained by player performance. It is approximately the point where we can trust that the data is a greater result of skill than noise.
Sounds like a good threshold, right? Fangraphs seems to think so. They display Carleton’s updated values on their own site.
There are plenty of detractors, however. Harry Pavlidis uses an r threshold of 0.5 in his own research. At Tom Tango’s suggestion, Derek Carty does the same. Tango himself has long argued for the use of 0.5. In Tango’s own words:
“Basically, Pizza sets the threshold at r=.70, whereas I set the threshold at r=.50. Why do I prefer mine? Because with my threshold, I can tell you exactly how much to regress the stats. It gives you extra information. In addition, I can explain it in English. If I set the OBP threshold at PA-210, then I can say: ‘If the player has 210 plate appearances, then his OBP is half real and half noise. Regress his OBP by 50% toward the mean.’”
This reasoning seems more like a convenience than actual evidence of Tango’s theory being better than Carleton’s. At the end of the day, these values are still semi-arbitrary. Considering both likely contain merit, let’s take a look at the hitter metric thresholds for both models.
Hitter Stabilization Thresholds | ||
Metric | r=0.5 | r=0.7 |
K% | 30 PA | 60 PA |
BB% | 75 PA | 120 PA |
HBP% | 275 PA | 240 PA |
1B% | 285 PA | 290 PA |
XBH% | 620 PA | 1610 PA |
HR% | 565 PA | 170 PA |
AVG | 270 AB | 910 AB |
OBP | 230 PA | 460 PA |
SLG | 235 AB | 320 AB |
ISO | 270 AB | 160 AB |
GB% | 30 BIP | 80 BIP |
FB% | 30 BIP | 80 BIP |
LD% | 280 BIP | 600 BIP |
HR/FB | 170 FBs | 50 FBs |
BABIP | 855 BIP | 820 BIP |
By Tango’s model, we can begin to analyze the Rockies from both a true outcomes perspective and a batted-ball profile perspective. However, I am going to play it safe (and lazy), and only analyze what Carleton’s model tells us we can conclude. At this point in the season, the only metric that seems to be usable is K%. Let’s dig deeper into the Rockies performance in that regard.
Rockies Strikeout Rates Comparison | ||||
Player | 2017 PAs | 2017 K% (As of 4/29) | 2016 K% | Career K% |
Charlie Blackmon | 112 | 17 | 15.9 | 15.7 |
Nolan Arenado | 104 | 13.5 | 14.8 | 14.6 |
DJ LeMahieu | 103 | 11.7 | 12.6 | 15.8 |
Mark Reynolds | 100 | 22 | 25.4 | 30.9 |
Trevor Story | 98 | 37.8 | 31.3 | 32.6 |
Carlos Gonzalez | 94 | 18.1 | 20.4 | 21.9 |
Gerardo Parra | 77 | 20.8 | 19.2 | 17.2 |
Average | 98.3 | 20.1 | 19.9 | 21.2 |
In aggregate, we see an ever so slight increase in K% from Rockies hitters that have achieved the 60 PA threshold compared to 2016. But the difference may not be of statistical significance. Of greater significance is the concerted efforts this team has made in improving contact rate over certain individual’s respective careers.
More specifically, I would like to point out two prime examples–DJ LeMahieu and Mark Reynolds. Both seem to have undergone a large change in approach prior to 2016. LeMahieu seems to have found a way to improve contact and power simultaneously. Reynolds, on the other hand, has been in the league for quite some time now. It’s amazing how he made a living as the single-season strikeout champ, only to reinvent himself for greater production at Coors Field.
Comments
Share your thoughts
Join the conversation
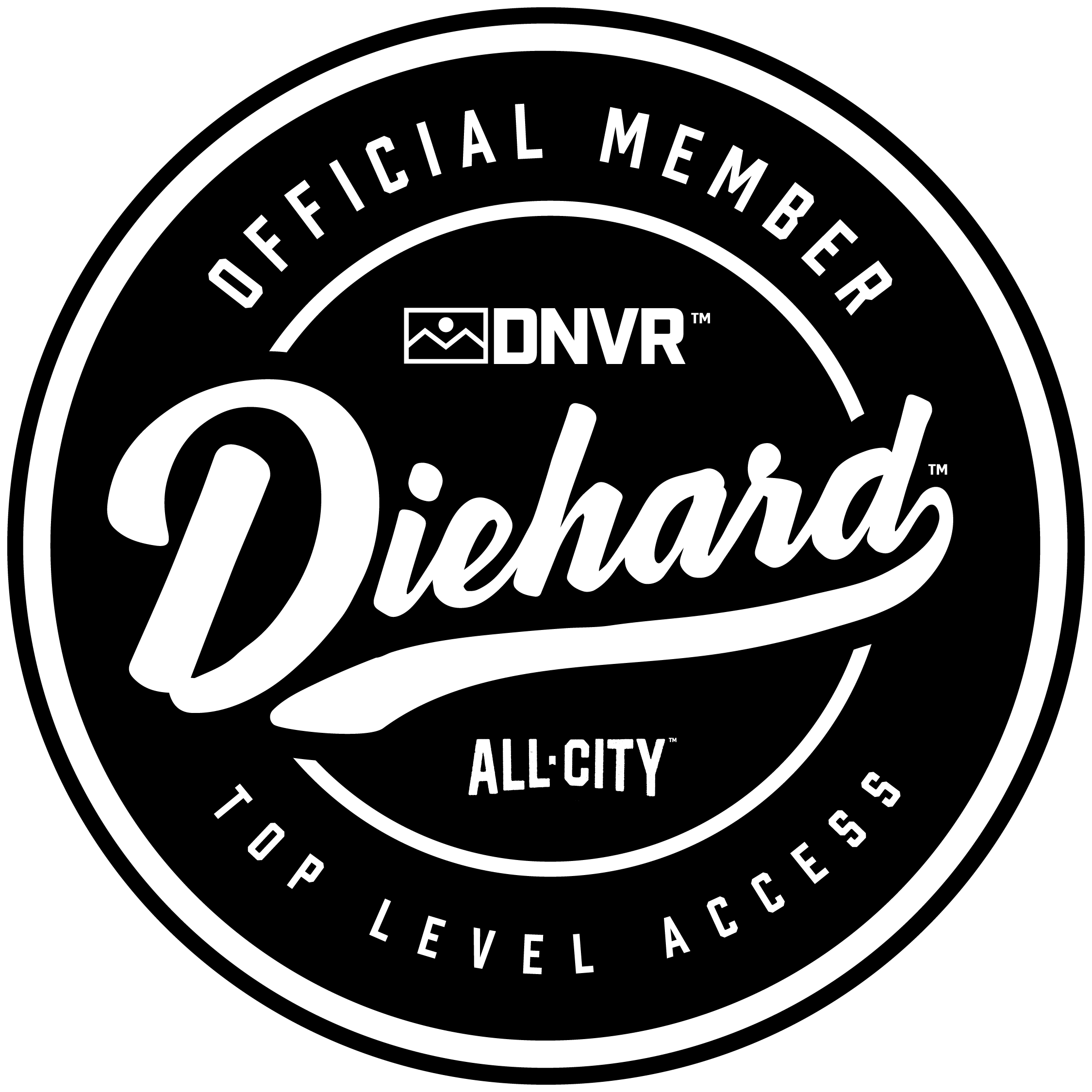
The Comment section is only for diehard members
Scroll to next article
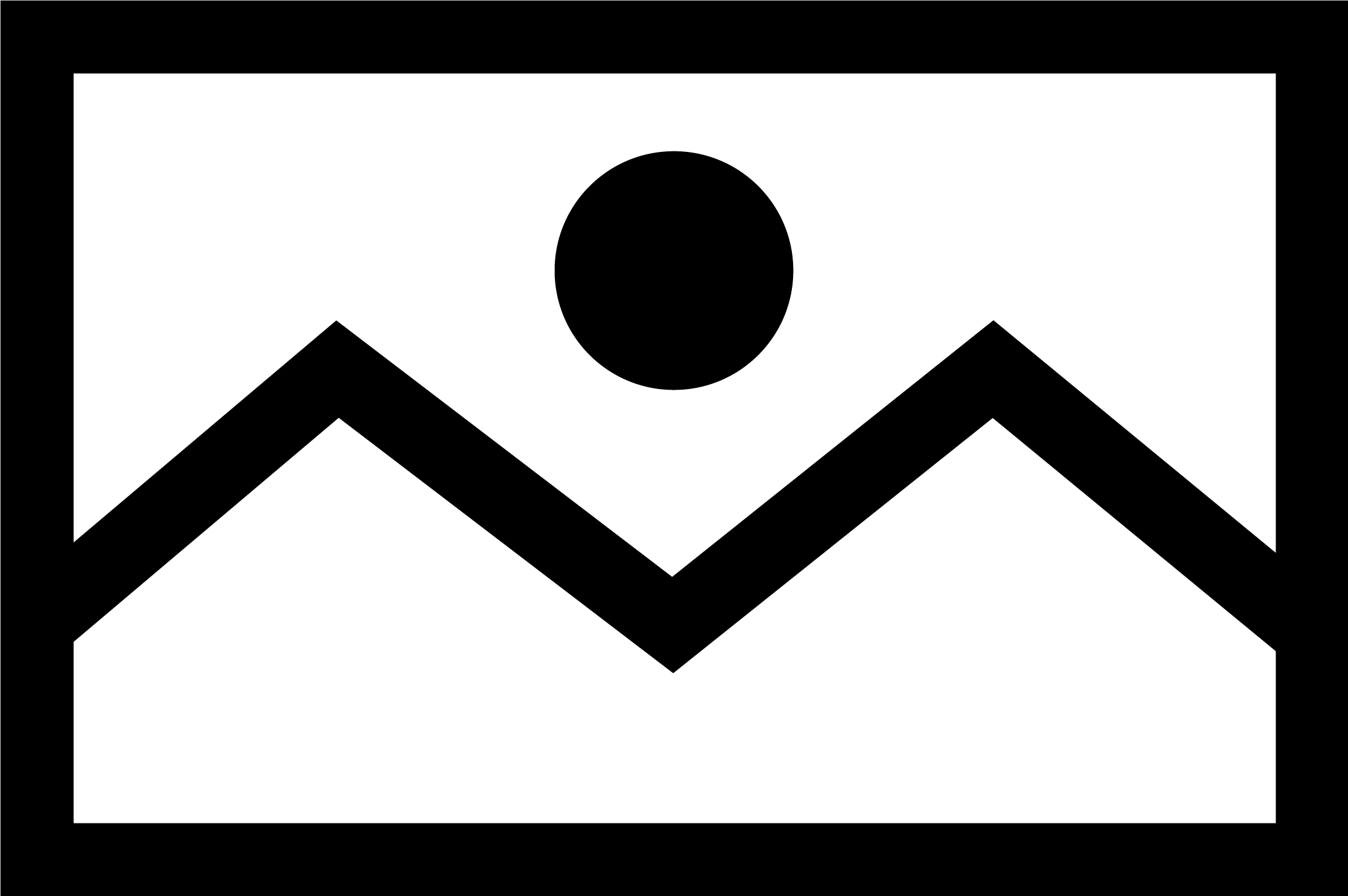